By Ben Wieland
In Game 2 of the 2021 NBA Finals, the Phoenix Suns took a commanding 2-0 series lead over the Milwaukee Bucks and seemed to be in the driver’s seat going forward. They knocked down 20 of their 40 three-point attempts, recorded 26 assists, and kept the Bucks at arm’s length en route to a 118-108 victory.
Beneath the surface, though, signs of trouble were lurking. ShotQuality’s game-analysis model — which aims to predict the outcome of a game based exclusively on the quality of shots taken by each team — estimated that, given the shot selection for each team, the Bucks would’ve won Game 2 approximately 88% of the time.
No one factor can explain the skepticism ShotQuality had towards what seems to be a convincing Suns victory. In fact, the model deviated from the actual outcome for a variety of reasons associated with each team:
Phoenix Suns
According to the ShotQuality model, the Suns overperformed their expected offensive outcome by about 15 points — they generated just 1.11 expected points per possession, below league average, but ended up scoring 118 points instead of the expected 103.3.
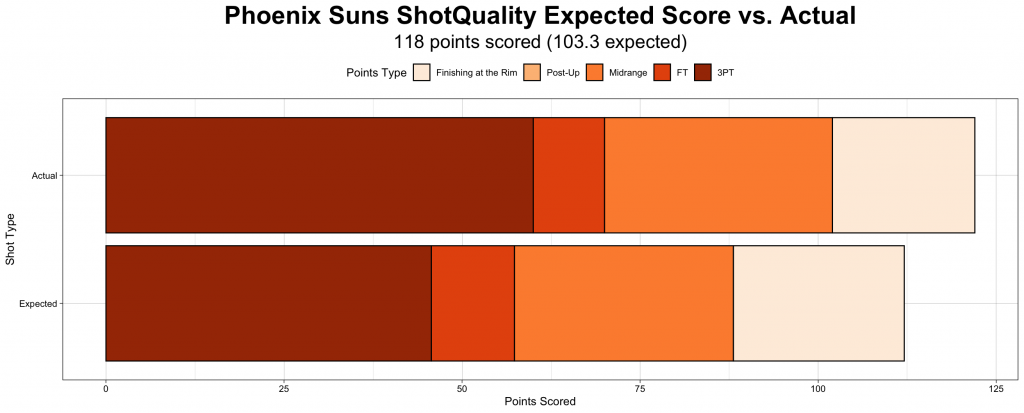
It might seem simple to conclude that a model like ShotQuality is naturally inclined to dislike teams like the Suns, who rely heavily on midrange jumpers to generate their offense. However, one important factor makes sure that this is not the case: the model takes into account who is taking a shot, not just where the shot was taken from.
For the 2021 season, the Phoenix Suns ranked second in the NBA in midrange attempt rate; they finished 24% of their possessions with a midrange jumper — generally not an optimal offensive strategy. However, since most of those shots were attempted by midrange maestros Chris Paul and Devin Booker, they also ranked first in the NBA in expected points per possession ending with a midrange jumper. Having good shooters take less-efficient shots can sometimes still lead to positive ShotQuality outcomes.
In Game 2, this was the case. The Suns scored 32 points on midrange jumpers, while the model projected that they would score 30.7 points. That 1.3-point difference isn’t nothing, but it also isn’t massive, and it certainly isn’t enough to explain why a team that won by double digits was given just a 12% win probability by the ShotQuality model.
The main culprit for the Suns’ offensive overperformance was, in fact, their three-point shooting success. They were absolutely generating good threes — they scored an average of 1.14 expected points per possession on their three-point attempts, which would’ve ranked in the top third of the NBA for three-point expected SQ PPP (another useful way to interpret this number is that, given the attempts taken by the Suns in Game 2, they would’ve been expected to shoot about 38% from beyond the arc).
However, even teams taking good shots in the NBA don’t usually shoot 50% from beyond the arc and knock down 20 triples. The Suns scored a total of 60 points on threes, compared to just 45.7 expected points. That 14.3-point difference is just about enough to account for the offensive overperformance the model observed. Every single major Suns shooter — Devin Booker, Chris Paul, Mikal Bridges, Jae Crowder, and Cam Johnson — overperformed their expected points scored.
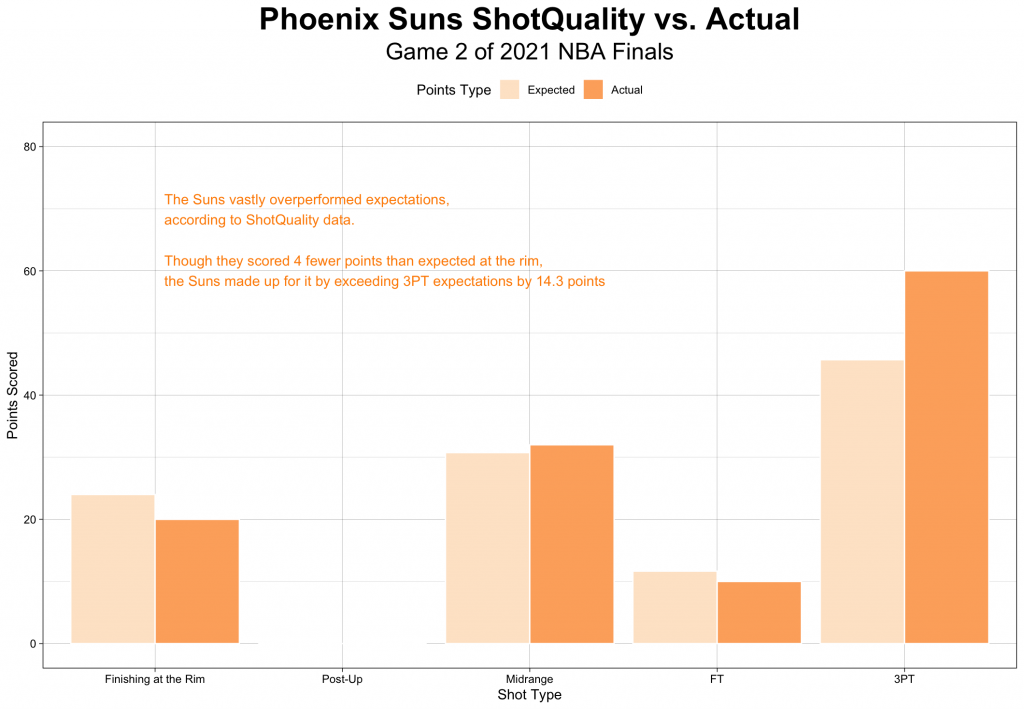
Milwaukee Bucks
On the other side of the floor, the Milwaukee Bucks put up just 108 points. However, the ShotQuality model loved their performance — it expected them to score 124.4 points on 1.24 PPP, which would’ve placed their offensive efficiency in the 91st percentile of the NBA.
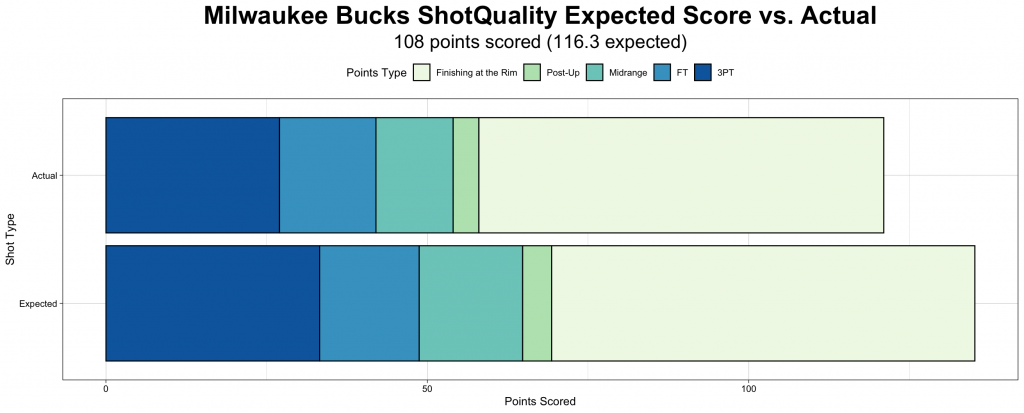
While the variance for the Suns could be mostly chalked up to their hot three-point shooting, the Bucks’ struggles were more multifaceted. In all five major shot types tracked by ShotQuality — shooting at the rim, from midrange, from three, at the line, and on post-ups — the Bucks underperformed their expected ShotQuality score.
Their three most drastic areas of issue were from three, from midrange, and at the rim. Beyond the arc, they shot just 9-31 from three, resulting in 27 points on 31 attempts. However, ShotQuality expected them to shoot just a little bit better than 11-31 and score 33.24 points beyond the arc, resulting in an underperformance of about six points.
From the midrange and at the rim, the story was about the same. The Bucks were expected to score 16.1 points on midrange jumpers, but ended up with just 12; they were expected to score 65.8 points at the rim, but actually finished with just 63.
As for assigning blame, eventual Finals MVP Giannis Antetokounmpko was not the culprit for this disappointing underperformance. He put up a monstrous 42 points and overperformed his expected SQ score by 1.25 points. Instead, it was his supporting cast letting the team down — Jrue Holiday and Khris Middleton combined for a measly 28 points and underperformed their expected SQ scores by a combined 13 points.
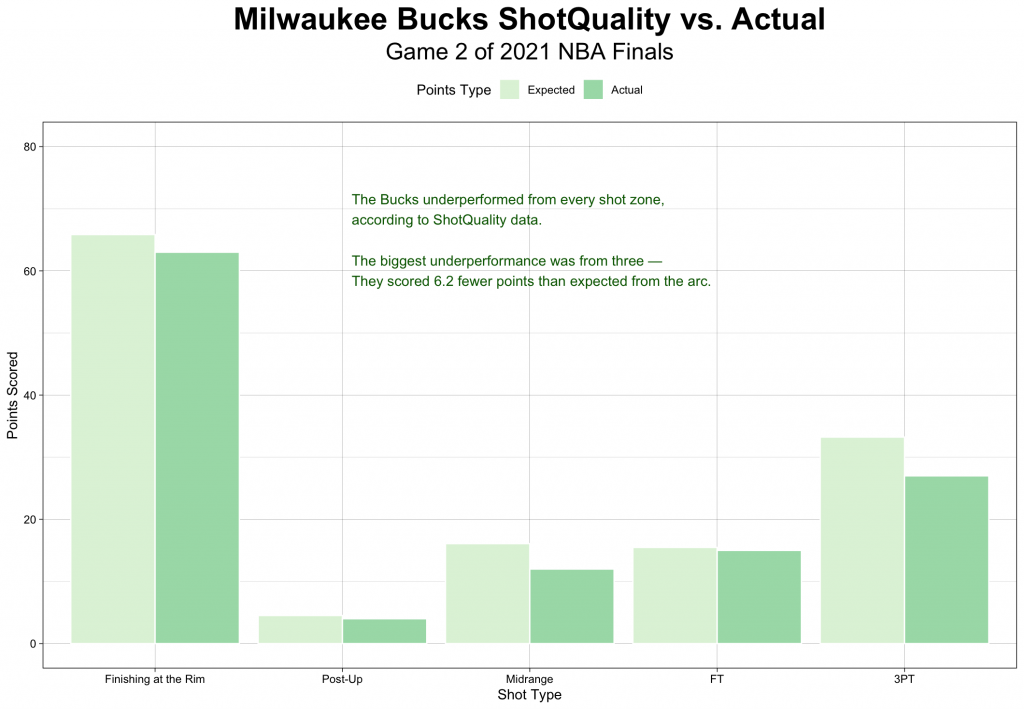
What Happened Next
By definition, an event that only has a 12% chance of occurring — like the Suns beating the Bucks in this game — happens 12% of the time, or approximately once in every eight events. This is improbable, but not impossible. Random variance is very real, and in one-game samples, it can have a massive effect.
Unfortunately for Phoenix, NBA playoff series aren’t one-off affairs (unlike their college counterparts, March Madness matchups). Teams play best-of-seven series to try and mitigate that random shooting variance and ensure that the “better” team eventually comes out on top.
In last year’s NBA Finals, that’s exactly what happened. After the Bucks fell behind 0-2 in the series, they flipped the script once the series moved to Milwaukee and reverse-swept the Suns, winning the Finals in six games.
As for the ShotQuality model, it accurately predicted the winner of five of the six Finals games. The lone exception was Game 2, where — for a variety of reasons explored above — the Suns overperformed their expected score by double digits and, at the same time, the Bucks underperformed their expected score by double digits. All things considered, though, an 83.3% accuracy rate isn’t too bad.